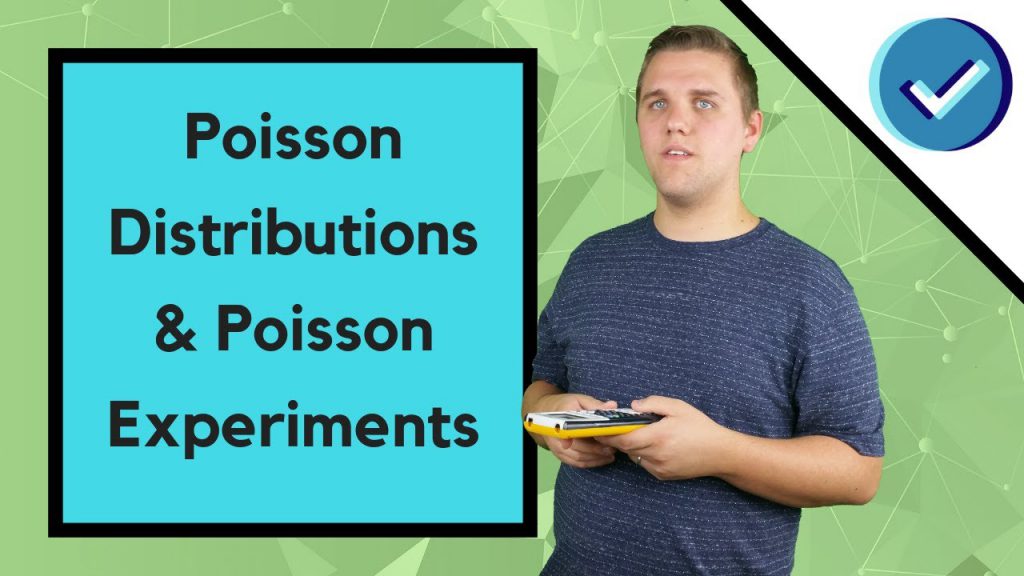
Think of a Poisson experiment example from your own area of interest. Describe how the selected experiment meets all the characteristics of a Poisson experiment. The Poisson distribution may also be used to approximate the binomial distribution. Explain this relationship with an example.
As a student of the Business Administration department, I would like to discuss the example of a Poisson Experiment in Business in this discussion forum assignment.
Imagine a retail store that receives customer orders online. Let’s focus on the number of orders the store receives during a fixed time interval of one hour. We’ll assume that the store’s average order rate is well-known and is approximately 10 orders per hour. Additionally, we’ll consider that the arrival of each order is independent of the time since the last order.
Now, let’s see how this example meets the characteristics of a Poisson experiment:
1. Number of Events: The events in this case are the customer orders placed within one hour. We are interested in counting how many orders occur in that fixed time interval.
2. Known Average Rate: We have an average rate of 10 orders per hour, which means, on average, the store receives about 10 orders during each hour.
3. Independence: The independence assumption holds true because the likelihood of a customer placing an order at any given moment is not influenced by previous order arrivals. Each order is considered a separate and independent event (CK-12, 2014).
Poisson Distribution and Approximation of Binomial Distribution:
The relationship between the Poisson distribution and the binomial distribution can be understood by considering the same example of a retail store that receives customer orders online. In this scenario, the store’s average order rate is known to be approximately 10 orders per hour.
The binomial distribution would be used to model the number of orders received by the store within a fixed number of trials, let’s say, in a 1-hour time frame. Each trial represents an opportunity for a customer to place an order, and the probability of success (i.e., placing an order) remains constant at 10 orders per hour.
For example, if we want to calculate the probability of exactly 5 orders in 1 hour using the binomial distribution, we would use the following formula:
P(X = 5) = (10 choose 5) * (0.5)^5 * (0.5)^(10-5)
However, as the number of trials becomes significantly large (e.g., over a long period of time) and the probability of success (i.e., placing an order) remains small (e.g., 10 orders per hour out of a large number of potential customers), we can approximate this situation using the Poisson distribution.
The Poisson distribution is often used to model the number of rare events occurring in a fixed interval when the events are independent and have a known average rate. In this case, the average rate is 10 orders per hour.
To approximate the binomial distribution using the Poisson distribution, we can use the following formula:
P(X = k) ≈ (e^(-λ) * λ^k) / k!
where λ (lambda) is the average rate of events (in this case, 10 orders per hour) and k represents the number of events (i.e., number of orders).
By applying the Poisson approximation, we can estimate the probability of a specific number of orders occurring within a given time period, such as 5 orders in 1 hour.
Using the Poisson distribution, the calculation would be:
P(X = 5) ≈ (e^(-10) * 10^5) / 5!
This approximation simplifies the calculation and becomes increasingly accurate as the number of trials (hours) becomes larger and the probability of success (order placement) becomes smaller.
References
CK-12. (2014, December 16). The Poisson Probability Distribution. Retrieved from https://www.ck12.org/book/ck-12-probability-and-statistics-advanced-second-edition/section/4.6/