The population of a culture of bacteria is modeled by the logistic equation P(t)= \frac{14,250}{1+29e^{-0.62t}. To the nearest tenth, how many days will it take the culture to reach 75% of its carrying capacity? What is the carrying capacity? What is the initial population for the model? Why a model like P(t)=P_0 \ e^{Kt} , where P_0 is the initial population, would not be plausible? What are the virtues of the logistic model?
1. To find out when the culture will reach 75% of its carrying capacity, we’ll use the same equation as before:
P(t) = 0.75 * Carrying Capacity
The carrying capacity is still 14,250 bacteria. Now, let’s solve for t:
0.75 * 14,250 = 14,250 / (1 + 29 * e^(-0.62 * t))
After some number crunching, we get:
e^(-0.62 * t) = 0.25 / 29
To solve for t, we’ll take the natural logarithm of both sides:
-0.62 * t = ln(0.25 / 29)
And finally, we find:
t = -ln(0.25 / 29) / 0.62 ā 17.4 days (rounded to the nearest tenth).
2. The carrying capacity for this bacterial culture remains at 14,250. That’s the maximum population the environment can sustain before the competition and limited resources kick in.
3. As we’ve calculated previously, the initial population (Pā) for this model is around 475 bacteria.
4. Now, let’s talk about why a model like P(t) = Pā * e^(Kt) wouldn’t be as plausible. Sure, it’s a simple exponential model, but it’s missing a crucial factor: the carrying capacity! In the real world, populations can’t just keep growing indefinitely. At some point, they reach a limit because of factors like limited resources, space, or competition for food and mates. The logistic model takes all of this into account, which makes it much more realistic for modeling biological populations.
5. The virtues of the logistic model are numerous:
a. **Realistic Population Growth**: The logistic model considers the carrying capacity, which is a fundamental concept in population ecology. It reflects the idea that populations can’t grow boundlessly and eventually stabilize.
b. **Accurate Predictions**: Since the logistic model accounts for limiting factors, it provides more accurate predictions of how populations will behave over time. This is super important for understanding how ecosystems function and for managing resources sustainably.
c. **Practical Applications**: The logistic equation is widely used in various scientific fields, such as ecology, biology, and epidemiology, to model and understand population dynamics. It’s a valuable tool for researchers and decision-makers alike.
d. **Insight into Sustainability**: By using the logistic model, we gain insights into the sustainability of populations and the consequences of exceeding the carrying capacity. It helps us find the balance between growth and resource conservation.
e. **Fits Empirical Data**: In many cases, real-world population data fits the logistic model better than the simple exponential one. This indicates that the logistic equation aligns well with observed population trends.
Graph will look as follows:
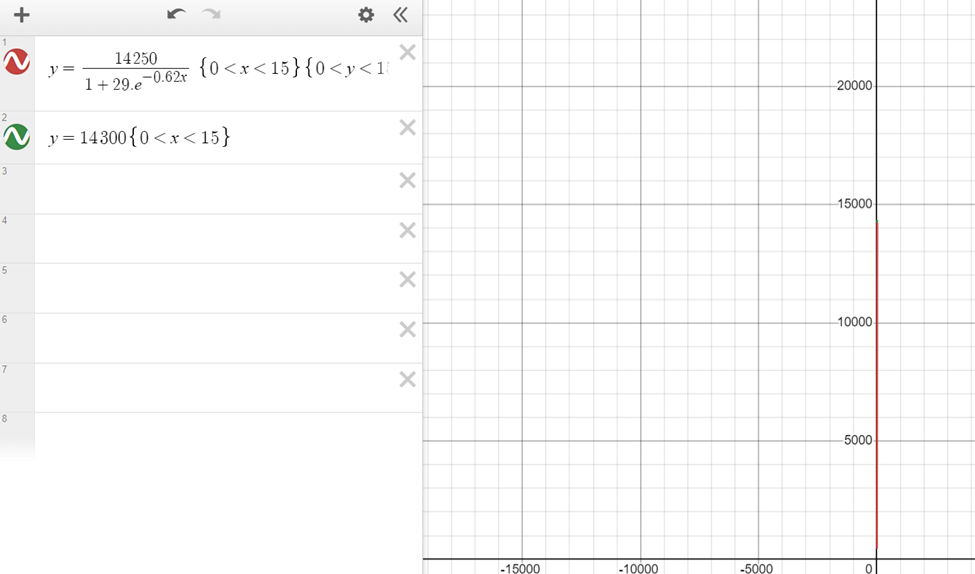
If we zoom in, it will look this way:
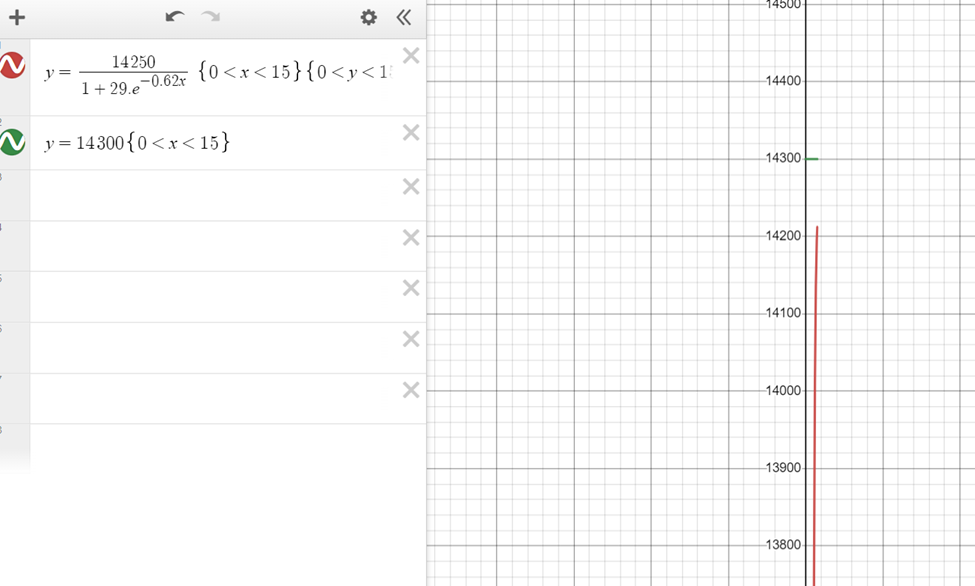